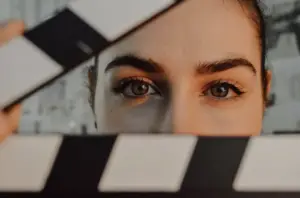
One of the hardest questions ever posed to humanity is a simple one – what should I watch? It’s the bane of media and commercial platforms everywhere, from Amazon to Spotify, and, seemingly, one without a solution. Taste is highly personal, after all, informed by several factors that might live deep within our DNA (or just our parents’ VHS collection).
Decision Paralysis
Making matters worse is something called decision paralysis, whereby many similar options turn our grey matter into a misfiring sludge. Decision paralysis happens when there are so many superhero movies, series, and animated shows (for example) to choose from that we default to watching The Simpsons for the fifteenth time. It’s an inability to choose when presented with overwhelming options.
Of course, as many of these decisions involve commercial products, marketers have an interest in forcing us to make one of these impossible choices. Netflix has a carousel of recommendations that tries to learn our tastes based on what we watch. Spotify is much the same. In fact, it pays for every media organization to feed us what they think we’ll like.
Source: Pexels
So far, so good. However, an issue arises with algorithmic recommendations when the user does something unexpected, something that, in reality, isn’t that odd at all – browsing a new genre. One wild evening sampling crime movies can derail a manicured list of anime recommendations for good, especially if the code was already struggling to find new suggestions.
AI is an obvious answer to this ultra-modern problem. Within gaming, another realm presenting unending choices, the NJ casino online app for Playstar utilizes machine learning to tailor players’ experiences. This “journey builder” uses an AI-driven platform that provides behavioral analysis to the platform.
Source: Unsplash
Progression Systems
Arguably, this tool is a riposte to the fact that recommendations have always been difficult in media, as the purest examples of genres are usually the oldest and, therefore, of less interest to modern audiences. The good news is that movies and TV shows generally don’t flirt as much with genre-bending as video games do, which makes the AI’s job a little easier.
There’s still an issue, involving inputs. Without useful data from the viewer (e.g. “I like action movies as long as they don’t have Tom Cruise in them”), recommendations are built from keywords, such as genres, dates, and languages.
Harvard Business Review suggests that a united approach to recommendations is most productive. Humans are better at targeting “experiential and sensory” elements. AI, on the other hand, is gifted at identifying “utilitarian and functional” things. Working together to combine these elements, they make the ideal recommendation engine.
The Centre for Economic Policy Research (CEPR) suggested that, again, with the right data, AI recommendations could become so powerful that they become anti-competitive, allowing the “good” ones to increase platform prices and shove the lesser robots out of the way.
AI still has much to learn but it’s showing potential as a recommendation engine, especially in the entertainment industry.